Digital solutions around us generate tremendous amounts of big data each day, and the immense computing power that is available to us enables the agriculture sector to benefit from the explosion of artificial intelligence in today’s age. While there is still much left to explore and achieve using AI in agriculture, it continues to alter our daily lives and change how we relate to and interact with the world around us.
In the banking sector, AI has predominantly enabled institutions to increase prosperity and growth for farmers and enterprises, provide better opportunities to enhance customer experience, and ensure more efficient management of compliance. AI-led solutions are also democratising financial services, ensuring better access to professional financial services. In recent years, AI has played a critical role in advancing cybersecurity with machine learning, thereby improving consumer protection and strengthening risk management. By and large, AI applications also contribute immensely to cost savings for enterprises, as per research that estimates potential savings of $447 billion by 2023.
Arguably, AI technology is potent and its applications are becoming more commonplace in several areas in the banking sectors, including decision-making (lending and credit scoring), risk management, fraud detection, anti-money laundering (AML), compliance, and personalisation of customers’ experiences, among others. It continues to strengthen global efforts to improve financial inclusiveness by providing many people with better access to financial products that they might not have had previously. However, it also brings to the fore questions and conversations surrounding the ethics of AI in agriculture. Some of them that need to be considered are discussed below.
Consumer Privacy And Data Security
While financial institutions gather data for business purposes and seek consent to do so in their long-winded T&Cs, consumers may not always read and understand the purpose for which the institution collects their personal data or the consequence of this data being analysed or shared with third parties. The challenge with AI in agriculture is that it can affect millions of smallholder farmers since a majority of them are either not educated enough or are tech-savvy to understand the implications of sharing personal data.
There are also questions concerning the ownership of the data that the AI technology will use. Does it belong to the consumer, the agribusiness that collects the data, or the third party that provides the AI solution? Does the enterprise also take adequate measures to protect against security breaches? When the farmer provides consent to gather, manage, and use personal data, can the bank use it in any way they please? Financial institutions will hence have to strike a right balance between their need for personal data and ensuring the farmer’s information privacy.
CropIn understands the importance of data sensitivity. Any confidential information or data that the client submits to CropIn belongs to the client and, under no circumstance, will we share the data with a third party, except with the written consent of the client. CropIn only uses this data for analytics to provide insights to the client. The data is also used to maintain, enhance, or add to the functionality of the service we provide and to personalise your experience.
Fairness and Bias
AI systems and machine learning models are designed to arrive at decisions based on socially-generated training data sets. To a considerable extent, these data sets reflect human biases and historical or social prejudices that have been well documented over the decades, especially against poorly-represented population groups. These inherent biases can hence prevent AI from being an ally to everyone. At a time when global organisations are working towards financial inclusiveness, particularly for those farmers who are under- or un-banked, there can be no margin for errors caused by AI bias.
While it may not be possible to eliminate human biases immediately, we can strive to create more unbiased algorithms based on data sets that are more inclusive and ensure fair and equal representation of all demographic groups. Additionally, AI algorithms can be used as tools to improve traditional human decision-making to ensure equal opportunities for all. Notably, the GDPR grants citizens of the European Union (EU) and European Economic Area (EEA) the right to not be subject to a decision (such as rejection of loan applications) solely based on automated data processing.
Accountability and Explainability
In traditional banking systems, the concerned personnel within the organisation were held accountable for their decisions. They provided individuals with reasons for rejecting a loan application and also adequate feedback for their actions. In contrast, AI systems arrive at conclusions without having to or being capable of explaining how or why they arrived at a particular result. How can these decisions then be clarified to farmers? Who is accountable for the decision-making process of an artificial entity and the outcome of such a process?
Similarly, explainability also plays a pivotal role in maintaining trust in technology. The workings of an AI system are complicated; it can be difficult for the bank or even machine learning designers to explain how or why the system arrived at a particular decision. In such an instance, who takes responsibility for AI-based decisions and actions? Helping farmers understand how the system generated the result, the data it has used, the assumptions it made and patterns it detected in the process will collectively allow individuals to trust AI applications better.
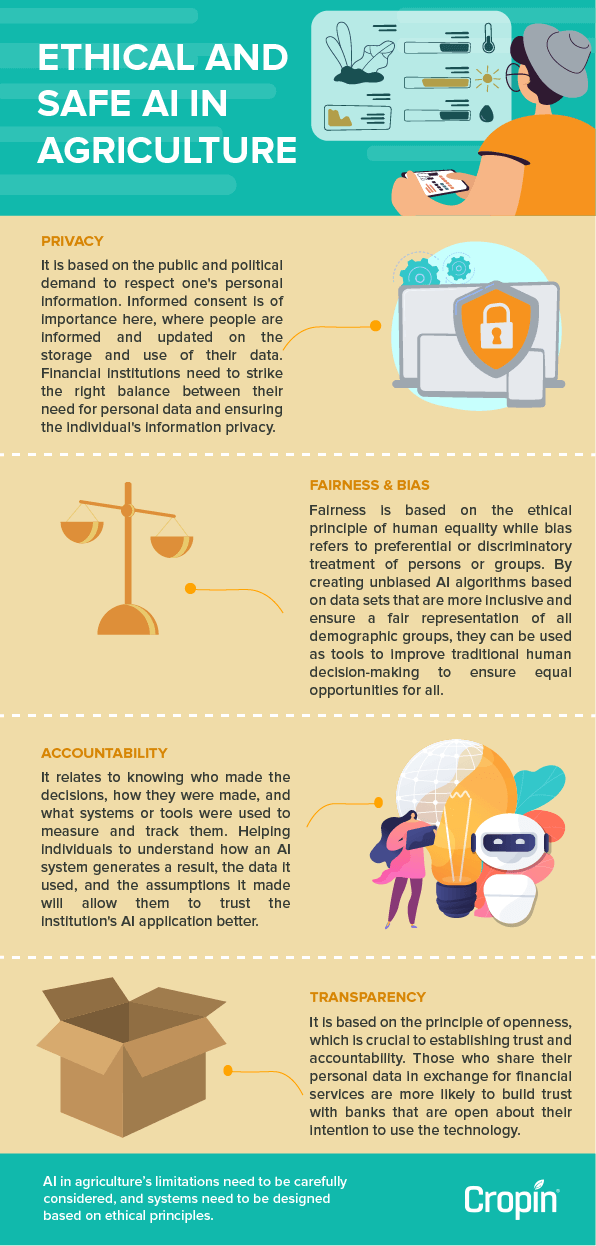
Transparency
AI-solution providers do not disclose the functioning of their algorithms for proprietary reasons, which can result in questions regarding the data that is used to train them and how the AI system makes a decision. In today’s digital age, given that customers, including farmers, provide personal data in exchange for financial services, they are more likely to build trust with banks that are open about their intention to use the technology as well as the system’s shortcomings.
CropIn’s Game-Changing AI-Led Solutions for Agri-Finance
AI in agriculture has a transformative role for credit and insurance providers, and has furthered the development of exciting new business models for the digital age. Financial institutions have already implemented AI systems to transform the borrowers’ experience by facilitating frictionless interactions. For farmers, they are beneficial in providing personalised recommendations and insights based on their previous transactions and credit history, as well as historical and predicted performance of their farmlands.
On the other hand, AI technology empowers institutions to prevent payment fraud, improve processes for AML, arrive at predictions that spot trends, identify risks, and economise on manpower. Using CropIn’s platform, loan officers and field sales executives can gather and verify farmers and plot information using their smartphones. This ground-level intelligence is then made available in a secure cloud platform in near-real-time for the bank official’s immediate use. The digitised data, along with easy-to-integrate APIs, also ensures hassle-free analysis and reporting when required.
With SmartRisk, lending institutions can leverage proprietary algorithms to identify areas under cultivation and monitor crop health up until harvest. Furthermore, banks can validate the information that farmers provide when applying for loans by comparing it with historical and predictive insights that SmartRisk derives from multiple sources of data. The platform also establishes the performance of every pixel to deliver regional (village/pincode/district/state) and plot-level intelligence at a fraction of the traditional cost and effort. It allows banks to underwrite loans more confidently using alternate agri-data and process credit to those farmers who display high assurance of loan repayment. This tech-enabled process empowers banks to manage loan delinquencies and NPAs more effectively, as well as enable timely collection of loans.